Understanding Machine Learning: A Comprehensive Guide
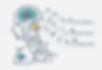
What is Machine Learning?
Machine Learning (ML) is a subset of artificial intelligence (AI) focused on the development of algorithms that enable computers to learn from and make predictions based on data. By analyzing patterns within data, ML algorithms can improve their performance over time without explicit programming. This technology underpins many modern applications, including image and speech recognition, recommendation systems, and autonomous vehicles. As the volume of available data continues to grow, machine learning has emerged as a powerful tool for tackling complex problems and enhancing efficiency across various industries. Its applications are vast, ranging from healthcare to finance, fundamentally changing how we interact with technology.
Types of Machine Learning
Machine Learning can primarily be divided into three categories: supervised learning, unsupervised learning, and reinforcement learning. In supervised learning, models are trained on labeled datasets, enabling them to predict outcomes for new, unseen data. Unsupervised learning, on the other hand, deals with unlabeled data and aims to uncover hidden patterns or groupings within it. Reinforcement learning is a dynamic approach where an agent learns by interacting with its environment, receiving feedback in the form of rewards or penalties. Each type of machine learning serves unique purposes and is applied in various scenarios, highlighting the versatility of the field.
Key Algorithms in Machine Learning
Several key algorithms form the backbone of machine learning applications. Among them, linear regression is often used for predicting continuous outcomes, while logistic regression serves classification tasks. Decision trees are popular for their interpretability and versatility, and ensemble methods, like random forests, improve accuracy by combining multiple models. Neural networks, inspired by biological systems, enable complex pattern recognition and form the basis of deep learning. Support vector machines (SVM) are crucial for classification issues, and clustering algorithms like K-means assist in grouping similar data points. Understanding these algorithms is essential for developing effective machine learning models.
The Role of Data in Machine Learning
Data is the cornerstone of machine learning; its quality and quantity directly impact the model's performance. Data preprocessing, which includes cleaning, normalizing, and transforming data, is crucial for effective training. The training set is used to teach the model, while the validation set fine-tunes its parameters, and the test set evaluates its performance on unseen data. Access to large and diverse datasets enhances the model's ability to generalize, while data bias can lead to skewed results. As businesses and researchers explore ML, a strong emphasis on data ethics and acquisition techniques will become increasingly important to ensure fair and accurate outcomes.
Challenges in Machine Learning
Despite its potential, machine learning faces several challenges that can hinder successful implementation. One significant issue is the need for substantial amounts of high-quality labeled data for supervised learning, which can be difficult to obtain. Additionally, overfitting—where a model learns noise instead of the underlying pattern—can lead to poor performance on new data. Interpretability is another concern, as many complex models, especially deep learning ones, function as "black boxes," making it challenging to understand how predictions are made. Addressing these challenges through innovative methods and tools is essential for advancing the field and ensuring trustworthiness in machine learning applications.
Future Trends in Machine Learning
The future of machine learning is promising, with several key trends expected to shape its evolution. The integration of ethical AI is likely to gain prominence, focusing on responsible practices and reducing bias in algorithms. Explainable AI (XAI) will enhance transparency, allowing users to understand model decisions better. As edge computing becomes more prevalent, machine learning models will increasingly run on devices, enabling real-time data processing and decision-making. Collaboration between humans and AI systems will expand, leading to hybrid models leveraging both human intuition and computational power. Additionally, advancements in natural language processing and computer vision will further enrich interactions between technology and users.
The Impact of Machine Learning
Machine learning is revolutionizing various sectors by enhancing efficiency, accuracy, and decision-making processes. Its applications contribute to groundbreaking developments across industries, from healthcare innovations that save lives to personalized customer experiences in retail. As research continues, machine learning will evolve, addressing current challenges and unlocking new possibilities. The interplay between data, algorithms, and computing power will drive advancements, creating systems that can adapt to real-world complexities.